Integrated Analytics and Visualization for Multi-Modality Transportation Data
Overview
This research project aimed to develop a data-driven approach for modeling cities, with a focus on pedestrian dynamics, which play a fundamental role in urban planning. It focused on detecting and counting objects such as pedestrians, cars, and bicycles in visual data sources that can provide insight into how people move around a city. The research team used an image database made up of tens of millions of images produced by Brooklyn-based start-up Carmera as its main data source.
Urban informatics is an important field that is attracting increasing attention from academia, government, and industry, and one particular area of interest in this field is in modeling the movement of people and goods around cities. A city model should represent information that allows the derivation of analytical methods to answer questions like: How do cities evolve? How can cities be compared, clustered, and distinguished?
Research Objectives
This project studied pedestrian dynamics, which is fundamental to improved urban planning, with applications in transportation network design, safety, and tax revenue. The researchers used tens of millions of images from the region of New York City from 2016 and 2017 and applied computer vision techniques to detect the pedestrians (and cars and bicycles) in each image, with the goal of building a spatio-temporal map of pedestrians for each sample location and time. The team recognized that such automatic detection of pedestrians is subject to errors, so they built simulations that can be used for performing high-confidence probabilistic detections of people. The goal was to model each mobile camera as a sensor and have them probabilistically detect people nearby.
Deliverables for this project include:
- Pedestrian model for parts of New York City
- A visualization and analytics tool able to display “merged” models
Personnel
Juliana Freire
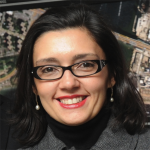
CO-PRINCIPAL INVESTIGATOR
Fabio Miranda
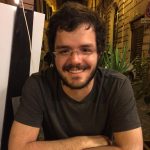
PH.D. CANDIDATE
Principal Investigator | Claudio Silva, New York University |
Funding Source | C2SMART: $69,304 NYU Fellowship (cost-share): $43,964 |
Total Project Cost | $113,268 |
USDOT Award # | 69A3551747124 |
Start and End Dates | 03/01/2018-05/31/2019 |
Implementation of Research Outcomes | This project: 1) Built a database of 40 million images, including performing data cleaning tasks; 2) Extracted pedestrian data using deep learning techniques; 3) Built coupled probabilistic simulation of sensors and pedestrians; 4) Developed analytics and visualization tools needed for exploration and validation of models and data. |
Impacts/Benefits of Implementation | This project produced advances both in overall computing methodology and the domain aspects of the work. The researchers developed new techniques for storing, mining, and visualizing terabytes of imagery data. The pedestrian dynamics models are of independent interest. |